The Impact of Machine Learning in Improving Diagnosis of Shoulder Injuries
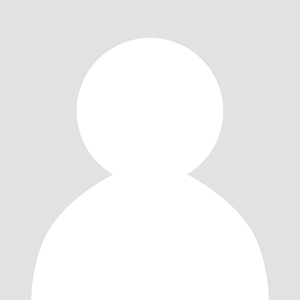
:format(webp)/cdn.mskdoctors.com/storage/2024/8/dVCafV5WuQKdQUTxUQzPNII1kmeZ4eHw.jpg)
Introduction
In the fast-evolving landscape of medical technology, Machine Learning (ML) is revolutionizing the way health professionals diagnose and manage shoulder injuries. This innovative approach not only enhances diagnostic accuracy but also optimizes patient outcomes, transforming the way shoulder pathologies are approached in both clinical and athletic settings.
Causes and Risk Factors
Shoulder injuries can result from a variety of causes, ranging from acute trauma (such as falls or direct impacts) to chronic stress (like repetitive overhead motions). Risk factors include engaging in high-impact sports, poor training techniques, inadequate recovery, and pre-existing conditions that weaken the shoulder's structural integrity. Machine learning helps identify subtle patterns in these injuries that might elude traditional diagnostic tools.
Common Symptoms and Diagnostic Methods
Symptoms of shoulder injuries typically include pain, restricted mobility, swelling, and a decreased range of motion. Traditional diagnostics involve physical examinations, MRI scans, X-rays, and ultrasounds. Machine learning enhances these methods by analyzing vast datasets to improve accuracy in identifying injury patterns and predicting recovery outcomes.
Immediate Actions and Emergency Care
Immediate management of suspected shoulder injuries should follow the RICE protocol—rest, ice, compression, and elevation. In cases of severe pain or visible deformities, immediate medical attention is crucial. Machine learning tools can aid in triaging these cases, prioritizing urgent cases based on predictive algorithms.
Prevention Strategies
Preventive measures for shoulder injuries involve strengthening exercises, proper technique training, and using protective gear during high-risk activities. Machine learning algorithms can analyze individual risk factors and tailor preventive exercises that maximize effectiveness in strengthening shoulder resilience.
Treatment Options
Treatment ranges from conservative methods like physical therapy and medication to more invasive procedures such as injections or surgery. ML algorithms assist in predicting which treatments are most likely to succeed based on individual patient profiles, thus personalizing care plans for optimal recovery.
Surgical Considerations
In cases where surgery is necessary, such as for severe rotator cuff tears or complete dislocations, machine learning models can help surgeons evaluate the potential benefits versus risks of surgical interventions and foresee possible complications, aiding in surgical planning and patient counseling.
Recovery and Rehabilitation
The rehabilitation process typically involves a combination of physical therapy, controlled activity resumption, and possibly further interventions. Machine learning optimizes this process by monitoring progress in real time and adjusting rehab protocols dynamically to individual response, thereby reducing recovery times and improving outcomes.
Special Considerations
Children and athletes might require specific strategies tailored to their unique physiological and functional demands. Machine learning can analyze demographic-specific data to optimize recovery protocols and prevent reinjury in these sensitive groups.
Returning to Normal Activities
Gradual reintroduction to normal or athletic activities is key to a successful recovery. Machine learning tools can predict when patients are ready to return to activity based on their recovery data, thus minimizing the risk of reinjury.
Potential Complications and Long-term Management
ML can identify patterns that indicate the risk of complications such as chronic instability or recurrent pain. Long-term management aided by machine learning can involve regular monitoring and adjustments to lifestyle or activity levels to prevent future injuries.
Frequently Asked Questions
Q: How does machine learning improve the diagnosis of shoulder injuries? A: ML analyzes large datasets to recognize patterns and predict outcomes more accurately than traditional methods, thus enhancing both diagnosis and personalized treatment planning.
Q: What are the benefits of using ML in managing shoulder injuries? A: Benefits include improved diagnostic accuracy, personalized treatment plans, optimized recovery strategies, and reduced risk of long-term complications.
Call to Action
For those experiencing shoulder pain or involved in managing shoulder injuries, embracing machine learning technologies offers a promising path to better outcomes. Contact health professionals who utilize these advanced diagnostic and management tools to ensure you are receiving the most informed, up-to-date care available. Visit [insert contact information or website] for more information or to schedule a consultation.
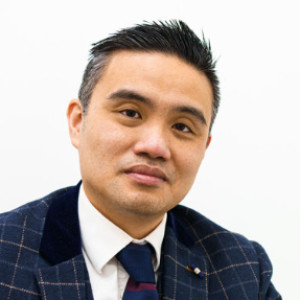
:format(webp)/cdn.mskdoctors.com/storage/2024/9/RxzKbUa0DHqR8y3uK9O4ef5qxmS0IP6A.jpg)
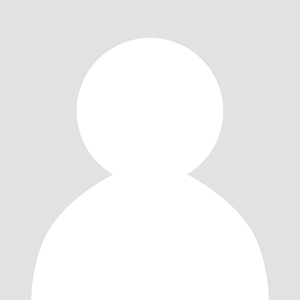
:format(webp)/cdn.mskdoctors.com/storage/2024/9/dLaOmik99XKAGZVfxzBuNPdCU9sWeeez.jpg)
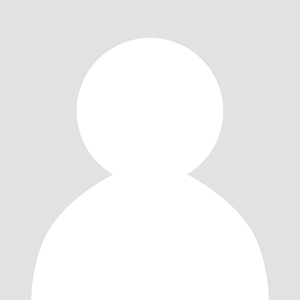
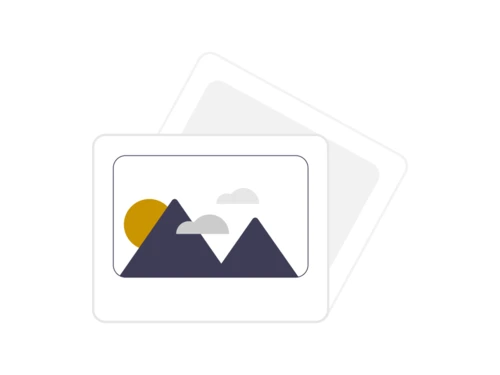
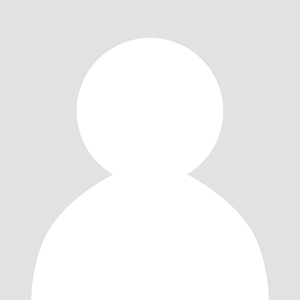